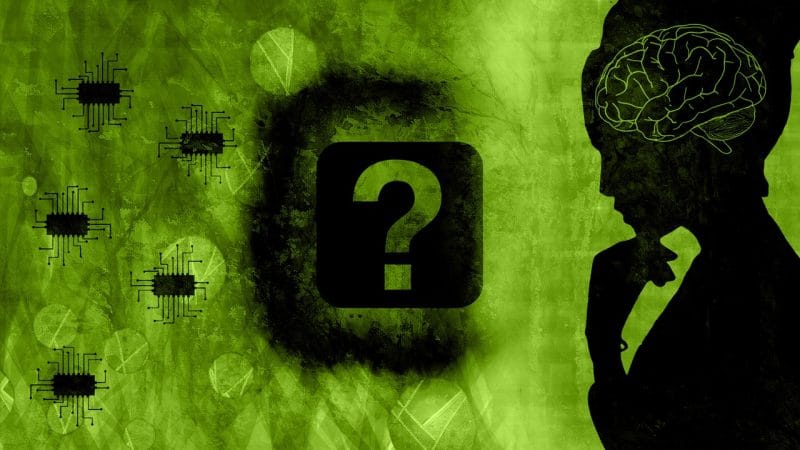
Artificial intelligence (AI) and machine learning (ML): What is the difference between them?
AI and ML have garnered substantial interest in the realm of technology for valid reasons. These technologies offer significant assistance to businesses in streamlining processes and extracting insights from data to make well-informed decisions. They drive progress across various industries, facilitating smarter operations, and have become indispensable tools for maintaining a competitive edge.
Notable applications of AI and ML include features such as facial recognition on smartphones, personalized online shopping experiences, virtual home assistants, and even medical diagnosis of illnesses.
The demand for professionals skilled in AI and ML is experiencing exponential growth. However, this rapid expansion presents challenges for organizations, including a lack of expertise, difficulties in understanding AI use cases, and concerns regarding data quality and scope.
Although the concept of AI and ML would have seemed inconceivable a few decades ago, they have now become increasingly commonplace in businesses. Despite their close relationship, it is crucial to recognize their significant differences.
Artificial Intelligence:
Artificial intelligence is a term that lacks precise definition, contributing to the confusion between AI and ML. In essence, AI refers to a system that exhibits intelligent behavior, although a precise definition remains elusive. Essentially, AI is an intelligent machine designed to mimic human-like behavior.
This behavior encompasses problem-solving, learning, planning, and other cognitive tasks accomplished through data analysis and identification of patterns to replicate human-like behavior.
Machine Learning:
On the other hand, machine learning is a subfield of AI. While AI represents the general notion of intelligence, machine learning involves machines learning knowledge and behaviors that may be challenging for humans to perform. In fact, machine learning has the potential to surpass human intelligence.
Primarily, this technology is employed to rapidly process vast amounts of data. It utilizes algorithms that evolve over time, continually improving their performance. For instance, a manufacturing plant could collect data from machines and sensors on its network in quantities far exceeding human capacity to process. In such cases, machine learning algorithms could identify patterns and anomalies that may indicate issues or inefficiencies.
Skill Requirements for AI and ML:
Given the close relationship between AI and ML, both demand qualified professionals to work effectively with these technologies.
AI professionals need to possess certain characteristics, including expertise in working with algorithms and employing analytical techniques. In-depth knowledge of data science, proficiency in Java programming, and familiarity with robotics are also essential.
Working with ML necessitates comprehensive training in applied mathematics, understanding the architecture of neural networks, and proficiency in natural language processing. Proficiency in various programming languages is also critical.
Interchangeable Use of AI and ML Terms:
The interchangeable use of the terms AI and ML arises from a lack of consideration for the distinctions between the two.
AI originated in 1956 and has undergone numerous changes and iterations since then. Initially, there was an expectation that AI would achieve human-level intelligence, but it became evident that such aspirations were not realistic. Coupled with limited funding, interest in AI waned.
Lately, some organizations have distanced themselves from the term AI, which had become associated with unrealistic expectations, and began using different names to describe their work. For instance, IBM referred to Deep Blue as a supercomputer and explicitly denied employing artificial intelligence, despite its utilization of AI techniques.
Similarly, Apple did not use the term AI in their latest Apple’s Worldwide Developers Conference on June 5th, 2023 for a few reasons. First, the company has a history of avoiding buzzwords, and AI is one that has been overused in recent years. Second, Apple prefers to focus on the practical benefits of its products, rather than the underlying technology. This is why they often use terms like “machine learning” or “natural language processing” instead of AI. Finally, Apple may be concerned about the negative connotations that AI has for some people. Some people worry that AI could become too powerful or that it could be used for malicious purposes. By avoiding the term AI, Apple may be trying to allay these fears and focus on the positive aspects of its products.
Overall, AI and ML have the potential to make our lives better in many ways. However, it is important to remember that these technologies are still evolving, and we need to be mindful of the potential risks as well as the benefits. By working together, we can ensure that AI and ML are used for good and that they help us build a better future for everyone.
Recent Posts
- Understanding the Difference Between Dataflows and Pipelines in Microsoft Fabric Data Factory
- How to Write AI Prompts That Actually Get You the Answers You Need?
- A comparison of popular AI APIs: OpenAI, Gemini, and Grok.
- The Transformative Impact of AI on the Insurance Industry
- Generative BI: Revolutionizing Business Intelligence with AI