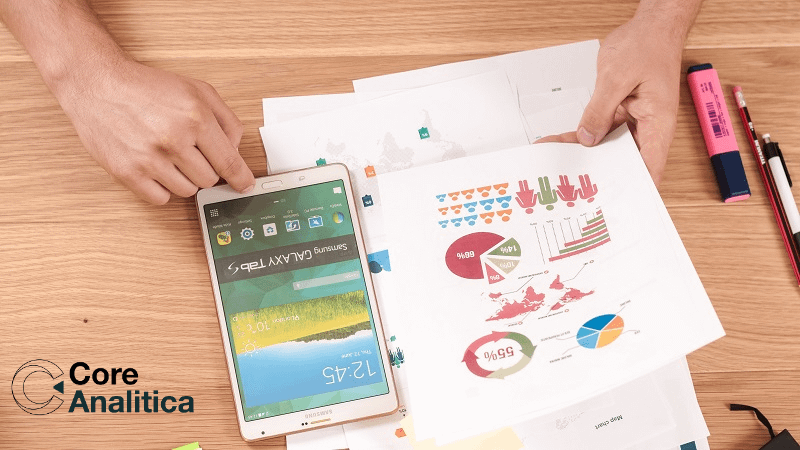
How to communicate effectively using stories and data (according to the book “Storytelling with Data”)
Data, especially numerical data, are elements that can be complex for readers. A good way to bring data to the real world and make it more understandable is storytelling. The art of telling a story in a way that conveys to recipients the ability to internalize, understand, and create personal meaning from it.
The prologue to the book Storytelling with Data: A data visualization guide for business professionals by Cole Nussbaumer Knaflic, mentions that having all the information in the world at your fingertips does not make communication easier: it makes it more difficult. The more information a person is dealing with, the harder it is to filter down to the most important bits.
The text comments that being able to tell stories with data is a skill that is becoming increasingly important in our data-rich world. An effective data visualization can mean the difference between success and failure when it comes to communicating the results of a study or simply getting your point across to your audience.
Data professionals should consider the following recommendations from Nussbaumer’s book when telling a story, because at the end of the day, the data should lead you to discover something to tell.
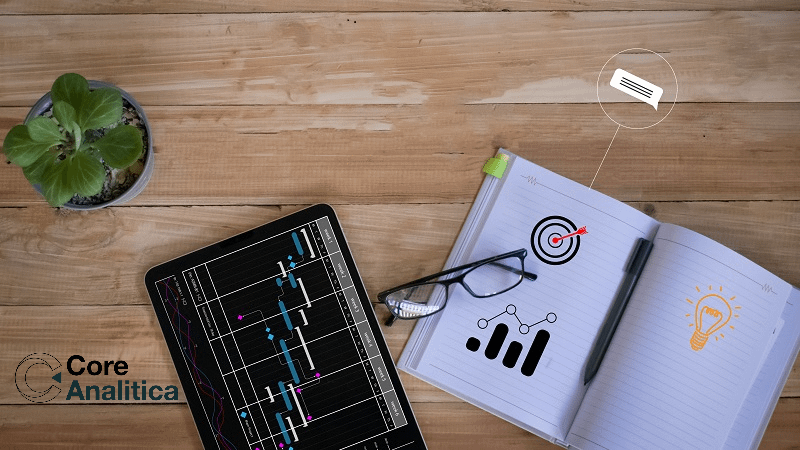
Storytelling with data
1. Understand the context
It is important to have context as a starting point for your story. You need to think about who you are communicating to and how your audience perceives you. This can help you identify what language you should use, what tone, what you need your audience to know about this story.
Storyboarding – a visual script of the content you plan to create – is perhaps the most important thing you can do to ensure that your communication is on point, as it establishes a structure and steps to follow. You can use: Shotbox, Storyboard Generator or Storyboard That.
2. Choose the correct visualization
Context is everything and will help you choose the right way to display your data. The first step should be to know what type of data you have. If you have few numbers maybe you don’t need a graph, sometimes a simple sentence is enough and much clearer.
The book dictates that “when there is just a number or two you want to communicate: use the numbers directly.” When there is more data to display, a table or graph is generally the way to go. One thing you need to understand is that people interact differently with these two types of images and in many cases, there is no single correct display; rather, there are often different types of images that could meet a given need.
3. Eliminate clutter
Nussbaumer mentions cognitive load as the mental effort required to learn new information. It’s what we do with the data we provide to an audience.
If we have a large amount of data and we do not communicate it in an organized way, the audience is not going to understand what we want them to understand. “Human brains have a finite amount of this mental processing power.” As information designers, we must be smart about how we use the brain power of our audience.
What we should avoid is processing that absorbs mental resources but does not help the audience understand the information. Also, if there is clutter, the story may seem more difficult than it really is.
4. Guide attention to where you want it
Here you need to think about how your audience observes and perceives the information. Design and visual tools can be used to create a visual hierarchy of elements to guide your audience through the information you want to communicate in the way you want them to process it.
One thing to keep in mind is that people tend to associate quantitative values with some, but not all, of the preattentive attributes (which are those that are processed in memory without our conscious action). For example, most people will consider a long line to represent a greater value than a short line. That’s one of the reasons bar charts are easy to read.
This is important because it tells us which of the attributes can be used to encode quantitative information (line length, spatial position, or to a more limited degree, line width, size, and intensity can be used to reflect relative value), and that should be used as categorical differentiators.
5. Think like a designer
When it comes to the form and function of our data visualizations, we first want to think about what we want our audience to be able to do with the data (function) and then create a visualization (form) that easily enables this.
You must be thinking: But I’m not a designer. “Stop thinking this way,” the book says, you can recognize intelligent design. By familiarizing ourselves with some commonalities and examples of great design, we instill confidence in visual instincts and learn some practical tips to follow and adjustments to make when things don’t feel right. For example, highlight what is important, eliminate distractions, create a clear visual hierarchy, use highly accessible design tools, and share it with others before publishing, so that they can give you feedback and comment on whether the message is clear or not.
Finally, in the world of data-driven decision-making, storytelling with data emerges as the bridge between raw information and actionable insights. As we’ve explored in this article, the art of crafting compelling narratives from data not only enhances understanding but also inspires action. Data storytelling harnesses the power of human connection and engagement, making complex information accessible and relatable to a wide range of stakeholders. As organizations continue their journey in the data-driven landscape, mastering the skill of storytelling with data will be the key to not just deciphering the numbers but shaping a future where data informs, guides, and empowers decision-makers at every level.
Tags:
Data AnalyticsRecent Posts
- A comparison of popular AI APIs: OpenAI, Gemini, and Grok.
- The Transformative Impact of AI on the Insurance Industry
- Generative BI: Revolutionizing Business Intelligence with AI
- Fabric Data Factory vs. Azure Data Factory: A Simple Comparison
- Understanding Fabric Warehouse: When to Choose It and How It Compares with Other Options in Fabric